- March 20, 2023
- Posted by: Michael Johnson
- Categories: AI, Business, Deep Learning, Machine Learning, Representation Learning
No Comments
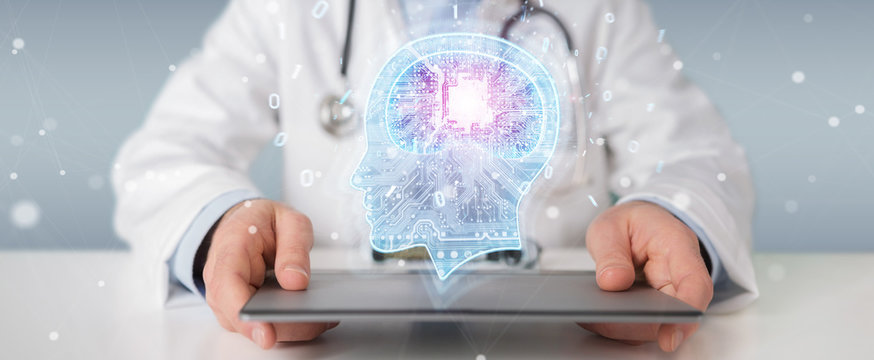
AI in Business Series: Healthcare Industry
What Business Sectors can AI help? Just about any business sector. With any business there are problems and challenges. If you can define what is causing issues with a business, you can apply tools to overcome these issues. AI has the potential to help many different business sectors, as the technology can automate and optimize a wide range of processes and tasks.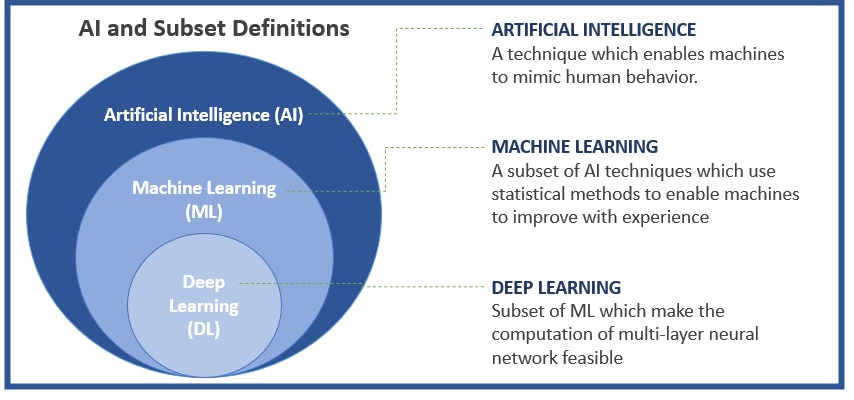
Finance/ Retail/ Marketing/ Insurance
- Finance: AI can be used to detect fraud, analyze market trends, and improve risk management.
- Retail: AI can be used to personalize customer experiences, optimize pricing and promotions, and improve supply chain management.
- Marketing: AI can be used to analyze customer data, target advertisements, and personalize marketing campaigns.
Energy/ Transportation/ Agriculture/ Manufacturing
- Energy: AI can be used to optimize delivery routes, predict maintenance needs, and improve safety.
- Transportation and Logistics: AI can be used to optimize delivery routes, predict maintenance needs, and improve safety.
- Agriculture: AI can be used to optimize crop management, improve yield prediction, and reduce waste.
Healthcare/ Pharma
.
- Healthcare: AI can be used to improve diagnosis, streamline patient data management, and assist with medical research.
- AI technology is used in each step of the drug designing procedure, which decreases the health hazards related to preclinical trials and also reduces the cost substantially. AI is an effective tool for data mining based on the huge pharmacological data and machine learning process.
Spotlight on Medical Sector
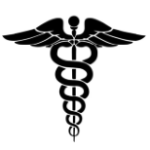
One prime example of an industry adopting Machine Learning is the medical sector. These are many ways Machine Learning is being used in healthcare to improve patient outcomes, increase efficiency, and support medical research. The use of Machine Learning in healthcare is a rapidly growing field, and the technology has the potential to transform the industry in many positive ways.
“Are Machine Learning devices in the medical industry actually saving lives?”
The answer is a big resounding yes! AI medical devices are saving lives. Machine Learning has the potential to revolutionize healthcare by improving patient outcomes, reducing healthcare costs, and increasing access to medical care.Machine Learning and Medical Devices
Overall, Machine Learning medical devices are already making a significant impact on healthcare, and their potential to save lives will only increase as the technology continues to evolve. Here are some examples of how Machine Learning medical devices are saving lives:
- Medical imaging: Machine Learning-powered medical imaging devices can detect anomalies in medical images such as X-rays, CT scans, and MRIs. By analyzing large amounts of imaging data, Machine Learning can help doctors to make more accurate and timely diagnoses, leading to better patient outcomes.
- Early detection: Machine Learning algorithms can be trained to analyze patient data and identify early warning signs of diseases such as cancer, heart disease, and Alzheimer’s disease. By detecting these conditions early, doctors can initiate treatment sooner, which can lead to better patient outcomes.
- Wearable devices: Machine Learning powered wearable devices such as smart watches and fitness trackers can monitor patients’ vital signs, track their activity levels, and detect changes in their health status. This can help patients to manage chronic conditions such as diabetes, heart disease, and asthma, and can alert healthcare providers to potential health issues before they become critical.
- Drug discovery: Machine Learning can be used to analyze large amounts of medical data to identify new drug candidates and develop personalized treatment plans. By accelerating the drug discovery process, Machine Learning can help to bring new treatments to market more quickly, potentially saving lives.
- Robotic surgery: Machine Learning powered robotic surgery systems can perform minimally invasive surgeries with greater precision and accuracy than human surgeons. By reducing the risk of complications and speeding up recovery times, these devices can help patients to recover more quickly and get back to their daily lives.
Machine Learning and Medical Applications
Machine Learning is being increasingly used in the healthcare industry to improve patient outcomes, increase efficiency, and support medical research. These are just a few examples of the ways that Machine Learning is being used in healthcare to improve patient outcomes, increase efficiency, and support medical research. The use of Machine Learning in healthcare is a rapidly growing field, and the technology has the potential to transform the industry in many positive ways.Healthcare Machine Learning Case Studies Here are a few case studies of how machine learning has been applied to healthcare:
Medical diagnosis: Machine Learning can be used to assist healthcare professionals in making more accurate and timely diagnoses by analyzing patient data and providing insights and recommendations.
- Imaging analysis: Machine Learning can be used to analyze medical images such as X-rays and MRI scans, helping to detect conditions and abnormalities that might otherwise be missed.
- Drug discovery: Machine Learning can be used to support the discovery and development of new drugs by analyzing large amounts of data from clinical trials and other sources.
- Personalized medicine: Machine Learning can be used to develop personalized treatment plans based on a patient’s unique genetic and health profile.
- Clinical decision support: Machine Learning can be used to provide real-time recommendations to healthcare professionals during patient consultations, helping to improve patient outcomes and quality of care.
- Predictive analytics: Machine Learning can be used to analyze patient data and make predictions about future health outcomes, such as the risk of disease or the likelihood of hospital readmission.
- Electronic health records: Machine Learning can be used to improve the management and analysis of electronic health records, helping healthcare professionals to access important patient information more quickly and efficiently.
Early Detection of Breast Cancer: Researchers at Google Health have developed a machine learning model that can identify breast cancer from mammograms with greater accuracy than human radiologists. The model was trained on a dataset of mammograms from over 76,000 women in the UK and over 15,000 women in the US. When tested on a separate dataset of mammograms, the model was able to reduce false negatives (missed cancers) by 9.4% and false positives (incorrectly flagged as cancer) by 5.7%.
- Predictive Analytics in ICU: Machine learning algorithms are used to predict which ICU patients are at risk of developing sepsis, a life-threatening condition caused by infections. The algorithm uses patient data such as vital signs, laboratory results, and other clinical information to predict the likelihood of sepsis. This information can be used to prioritize interventions and improve patient outcomes.
- Personalized Medicine: Machine learning algorithms are used to analyze genetic data and predict a patient’s response to different drugs. By analyzing large datasets of genetic information and clinical outcomes, researchers can identify genetic markers that are associated with drug response. This information can be used to personalize treatment plans and avoid prescribing drugs that are unlikely to be effective.
- Disease Diagnosis: Machine learning algorithms can be used to analyze medical images and diagnose diseases such as Alzheimer’s disease, Parkinson’s disease, and multiple sclerosis. By analyzing patterns in medical images, machine learning algorithms can identify subtle changes that are not visible to the human eye. This can help physicians make earlier and more accurate diagnoses.
- Hospital Readmission Prediction: Machine learning algorithms can be used to predict which patients are at risk of being readmitted to the hospital after discharge. By analyzing patient data such as demographics, medical history, and medication use, machine learning algorithms can identify factors that increase the risk of readmission. This information can be used to develop targeted interventions to reduce the risk of readmission and improve patient outcomes.
Video: Microsoft “AI for Health” on AI Technologies in the Healthcare Industry
Summary: 2:30 min video – Microsoft is expanding on its existing AI for Good initiative by launching the AI for Health program. AI for Health empowers researchers and organizations with AI to advance the health of people and communities around the world.